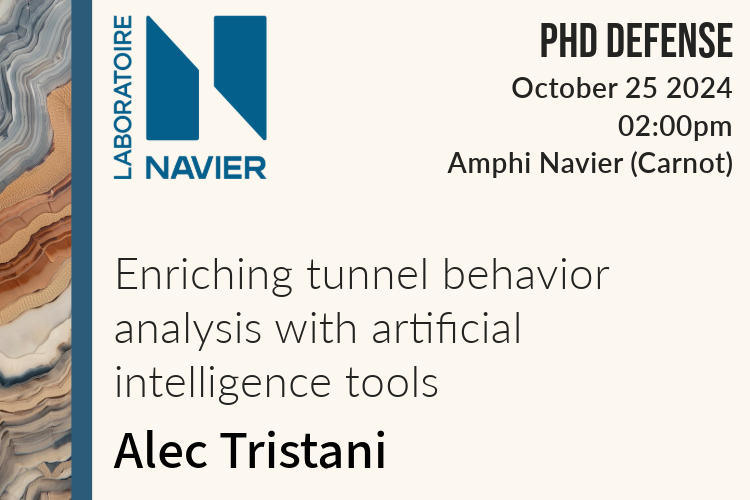
PhD Defense – Alec Tristani
- Post by: Mathias Lebihain
- 22 October 2024
- No Comment
Alec Tristani, PhD student in the Geotechnics team, will defend his PhD thesis entitled “Enriching tunnel behavior analysis with artificial intelligence tools” on Friday the 25th of October starting at 2pm in the Navier amphitheatre.
Composition of the jury:
- Chloé Arson – Cornell University – Reporter
- Daniela Boldini – Sapienza University of Rome – Reporter
- Georg Anagnostou – ETH Zurich – Examiner
- Jean-Michel Pereira – École des Ponts – Examiner
- Jean Sulem – École des Ponts – Thesis Director
- Lina-María Guayacán-Carrillo – École des Ponts – Co-Supervisor
- Didier Subrin – Centre d’Études des Tunnels – Guest
- Huy Tran-Manh – Itasca Consulting – Guest
Abstract
Show the abstract
Tunnel design relies on multiple approaches. Analytical and empirical formulations are reliable and useful for rapid estimations. Numerical modeling enables to overcome too restrictive assumptions and may be required in complex situations. However, additional efforts are needed to improve the robustness of these approaches. Especially, the time-dependent effects need to be better described to evaluate the long-term behavior of tunnels and there is a need for new constitutive models. Furthermore, to analyze the increasing amount of measurements collected during tunnelling, new techniques must be developed. Machine learning appears to be a promising field as previously unknown relationships between observations and measurements can be established. Thus, in this thesis, analytical, numerical, and data-driven approaches are emphasized to analyze the behavior of tunnels, both in the short term and in the long term. By combining these methods, a global framework for tunnel design is developed.
On the one hand, an original approach is presented for the back-analysis of convergence measurements in deep tunnels to determine the constitutive parameters of the surrounding rock mass. Since time-dependent deformations can result from both the advance of the face and the delayed response of the ground, the two effects must be considered during excavations. To that end, new analytical solutions are derived by assuming an unlined circular tunnel excavated in a homogeneous isotropic ground under an initial isotropic stress field and assuming a fractional viscoelastic plastic behavior. Combining the closed-form solution that takes into account the progressive face advance and an empirical approach, convergences are back-analyzed based on a least-squares optimization method to calibrate the constitutive parameters of the ground. The presented process aims to characterize the short-term and long-term behavior of tunnels and offers the advantage of being applicable during the excavation phase as soon as convergence measurements are available. The method is illustrated by two case studies related to the Fréjus road tunnel and the Saint-Martin-la-Porte access gallery (SMP2). Additionally, the ground-lining interaction is studied through the development of an analytical solution for a lined tunnel excavated in a fractional viscoelastic ground.
On the other hand, the potential use of artificial intelligence is explored. To this end, three machine learning tools are built based on synthetic data to predict both the short-term and the long-term behavior of the ground. The first model aims to assess the support pressure and the radial displacement at equilibrium in the lining and the radial displacement that occurs close to the face at the installation distance of the support. The second model computes the extrusion occurring at the core of the face considering an unlined gallery. The last model includes the time-dependent effect in the analysis to evaluate the ground-lining interaction in the long term. In particular, the bagging method is applied to neural networks to enhance generalization. Good performance is obtained using relatively scarce datasets. The modeling of the surrogate models is explained from the creation of the synthetic datasets to the evaluation of their performance and their limitations are discussed. In practice, these machine learning tools should be helpful in the field during the excavation phase.